

Chenyi Kuang
PhD student, Rensselaer Polytechnic Institute
About Me
Hello! I’m Chenyi Kuang. Currently I'm a PhD student in ECSE (Electrical, Computer and System Engineering) Department of Rensselaer Polytechnic Institute, under the supervision of Prof.Qiang Ji. My research mainly focus on computer vision tasks in the area of human facial behavior analysis using the tools of deep learning models and 3D geometric models. More concretely, my research is targeting at reconstructing two facial motions: the non-rigid 3D facial motion in terms of facial action units (FAUs) and the rigid 3D eyeball motion, for facial expression recognition and eye gaze tracking.
Research Area: Computer Vision, Deep Learning, 3D Reconstruction and Modeling.
Bio
Education
Research Project
Publication
AU-aware 3D Face Reconstruction through Personalized AU-specific Blendshape Learning
We present a multi-stage learning framework that recovers AU-interpretable 3D facial details by learning personalized AU-specific blendshapes from images. Our model explicitly learns 3D expression basis by using AU labels and generic AU relationship prior and then constrains the basis coefficients such that they are semantically mapped to each AU. Our AU-aware 3D reconstruction model generates accurate 3D expressions composed by semantically meaningful AU motion components. Furthermore, the output of the model can be directly applied to generate 3D AU occurrence predictions, which have not been fully explored by prior 3D reconstruction models.
More details can be found at: https://sites.ecse.rpi.edu/~cvrl/3DFace_Eye/3DFace.html
[1] Kuang, Chenyi, Zijun Cui, Jeffrey O. Kephart, and Qiang Ji. "AU-Aware 3D Face Reconstruction through Personalized AU-Specific Blendshape Learning." In European Conference on Computer Vision, pp. 1-18. Springer, Cham, 2022. paper
Towards an accurate 3D deformable eye model for gaze estimation
We present a method for constructing an anatomically accurate 3D deformable eye model from the IR images of eyes and demonstrate its application to 3D gaze estimation. The 3D eye model consists of a deformable basis capable of representing individual realworld eyeballs, corneas, irises and kappa angles. To validate the model’s accuracy, we combine it with a 3D face model (without eyeball) and perform image-based fitting to obtain eye basis coefficients The fitted eyeball is then used to compute 3D gaze direction. Evaluation results on multiple datasets show that the proposed method generalizes well across datasets and is robust under various head poses.
More details can be found at: https://sites.ecse.rpi.edu/~cvrl/3DFace_Eye/3D_eye.html
Chenyi Kuang, Jeffrey Kephart, and Qiang Ji. Towards an accurate 3D deformable eye model for gaze estimation. Towards a Complete Analysis of People: From Face and Body to Clothes (T-CAP Workshop at ICPR), 2022 paper
AU-Aware Dynamic 3D Face Reconstruction from Videos with Transformer
We present a framework for dynamic 3D face reconstruction from monocular videos, which can accurately recover 3D facial geometrical representations for facial action unit (AU). Specifically, we design a coarse-to-fine framework, where the ”coarse” 3D face sequences are generated by a pre-trained static reconstruction model; and the ”refinement” is performed through a Transformer-based network. We design 1) a Temporal Module used for modeling temporal dependency of facial motion dynamics; 2) an Spatial Module for modeling AU spatial correlations from geometry-based AU tokens; 3) feature fusion for simultaneous dynamic facial AU recognition and 3D expression capturing. Experimental results show the superiority of our method in generating AU-aware 3D face reconstruction sequences both quantitatively and qual- itatively.
Kuang, Chenyi, Jeffrey O. Kephart, and Qiang Ji. "AU-Aware Dynamic 3D Face Reconstruction From Videos With Transformer." Proceedings of the IEEE/CVF Winter Conference on Applications of Computer Vision. 2024. paper
Interaction-aware Dynamic 3D Gaze Estimation in Videos
We propose a novel method for dynamic 3D gaze estimation in videos by utilizing the human interaction labels. Our model contains a temporal gaze estimator which is built upon Autoregressive Transformer structures. Besides, our model learns the spatial relationship of gaze among multiple subjects, by constructing a Human Interaction Graph from predicted gaze and update the gaze feature with a structure-aware Transformer. Our model predict future gaze conditioned on historical gaze and the gaze interactions in an autoregressive manner. We propose a multi-state training algorithm to alternately update the Interaction module and dynamic gaze estimation module, when training on a mixture of labeled and unlabeled sequences. We show significant improvements in both within-domain gaze estimation accuracy and cross-domain generalization on the physically-unconstrained gaze estimation benchmark.
Kuang, Chenyi, Jeffrey O. Kephart, and Qiang Ji. "Interaction-aware Dynamic 3D Gaze Estimation in Videos." In NeuRIPS 2023 Workshop on Gaze Meets ML. 2023. paper
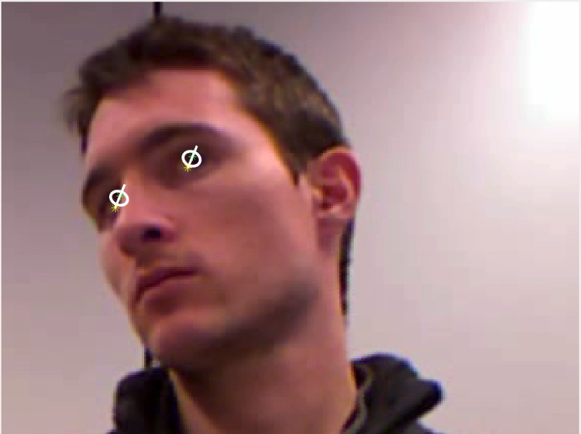
Model-based 3D Gaze Estimation
This work is under-review.
Physics-informed 4D face reconstruction
This work is undergoing.
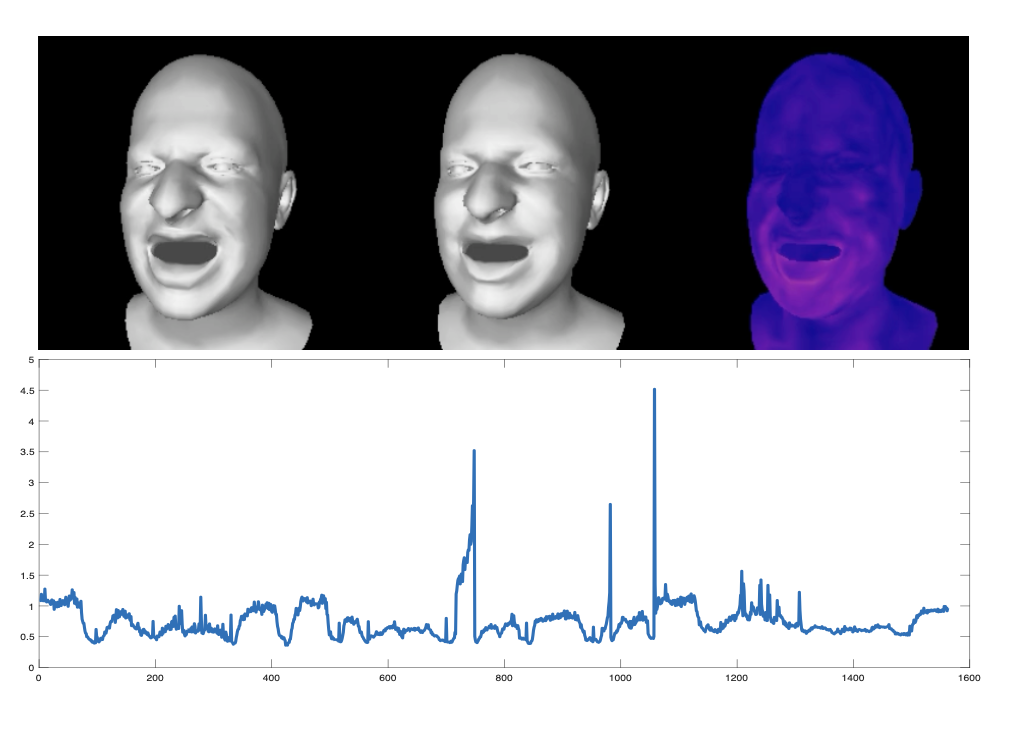
Contact
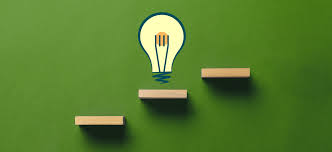