Summary
| Background
| Methods | Results | Publications
Model-Based Automated Segmentation
|
The
goal of this research is to improve the accuracy and efficiency of volume
segmentation by developing an automated segmentation method. In particular,
we investigate how to systematically incorporate prior knowledge to make
robust segmentation of difficult electron tomography images. Due to the
inherent difficulties in sub-cellular images and the limitations of the
existing methods, the successful segmentation of microtubules and the
filamentous plus-ends also requires the combination of multiple methods
into the segmentation. To this end, we propose a model-based automated
approach to extracting the microtubules and the plus-ends with a
coarse-to-fine scale scheme consisting of volume
enhancement, model-based microtubule
segmentation and probabilistic plus-end
tracing.
|
|
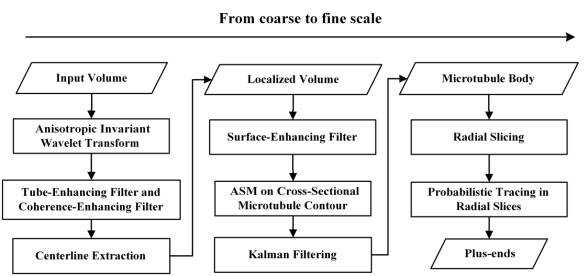
|
|
In volume enhancement, we fully exploit the
local and global geometric properties of the tubular objects. An invariant and
anisotropic wavelet filtering is first presented to globally enhance the
elongated structures. This is followed with 3D geometric filters that
locally accentuate the tubular objects and diminish objects of other
morphologies. For the segmentation of microtubules in the enhanced volume,
an improved statistical shape model is first built to capture the shape of
the cross-sectional contours of the tubular objects. It is then combined
with Kalman filtering to impose smoothness constraint along the longitudinal
direction of the tubes. For tracing the finer structures connected with the
tubular objects, we propose a probabilistic tracing method by integrating
both the shape and appearance knowledge of the curvilinear features into a
particle filter framework.
|
Summary | Background | Methods | Results | Publications
|